VibroSense: Recognizing Home Activities by Deep Learning Subtle Vibrations on an Interior Surface of a House from a Single Point Using Laser Doppler Vibrometry
Published on Proceedings of the Association for Computing Machinery on Interactive, Mobile, Wearable and Ubiquitous Technologies (IMWUT)/Ubicomp’20
Wei Sun, Tuochao Chen, Jiayi Zheng, Zhenyu Lei, Lucy Wang, Benjamin Steeper, Peng He, Matthew Dressa, Feng Tian, Cheng Zhang
Selected Media Coverage: Cornell Chronicle, Engadget, NewAtlas , Yahoo, TechExplore, PhysicsWorld, The Engineer, Hackster .
Spectrogram of the vibrations for 18 home appliances
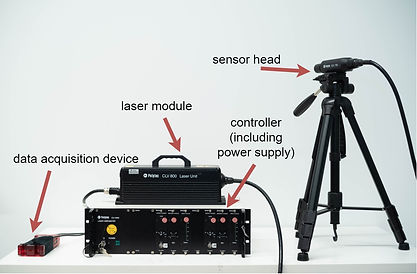
Smart homes of the future are envisioned to have the ability to recognize many types of home activities such as running a washing machine, flushing the toilet, and using a microwave. In this paper, we present a new sensing technology, VibroSense, which is able to recognize 18 different types of activities throughout a house by observing structural vibration patterns on a wall or ceiling using a laser Doppler vibrometer. The received vibration data is processed and sent to a deep neural network which is trained to distinguish between 18 activities. We conducted a system evaluation, where we collected data of 18 home activities in 5 different houses for 2 days in each house. The results demonstrated that our system can recognize 18 home activities with an average accuracy of up to 96.6%. After re-setup of the device on the second day, the average recognition accuracy decreased to 89.4%. We also conducted follow-up experiments, where we evaluated VibroSense under various scenarios to simulate real-world conditions. These included simulating online recognition, differentiating between specific stages of a device's activity, and testing the effects of shifting the laser's position during re-setup. Based on these results, we discuss the opportunities and challenges of applying VibroSense in real-world applications.
Data Acquisition Hardware Set
